Spaces:
Running
Running
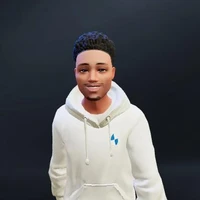
Integrate WhisperX for improved audio transcription and add real-time conversation support: update requirements to include WhisperX, refactor voice cloning to utilize WhisperX, implement WebSocket endpoints for real-time audio processing, and enhance audio transcription capabilities with alignment options.
be2a132
""" | |
API routes for the CSM-1B TTS API. | |
""" | |
import os | |
import io | |
import base64 | |
import time | |
import tempfile | |
import logging | |
import asyncio | |
from enum import Enum | |
from typing import Dict, List, Optional, Any, Union | |
import torch | |
import torchaudio | |
import numpy as np | |
import whisperx | |
from fastapi import APIRouter, Request, HTTPException, BackgroundTasks, Body, Response, Query, UploadFile, File | |
from fastapi.responses import StreamingResponse, JSONResponse | |
from app.api.schemas import SpeechRequest, ResponseFormat, Voice | |
from app.model import Segment | |
from app.api.streaming import AudioChunker | |
from app.prompt_engineering import split_into_segments | |
# Set up logging | |
logger = logging.getLogger(__name__) | |
router = APIRouter() | |
# Mapping of response_format to MIME types | |
MIME_TYPES = { | |
"mp3": "audio/mpeg", | |
"opus": "audio/opus", | |
"aac": "audio/aac", | |
"flac": "audio/flac", | |
"wav": "audio/wav", | |
} | |
# WhisperX model cache for reuse | |
whisperx_model = None | |
whisperx_model_lock = asyncio.Lock() | |
def get_speaker_id(app_state, voice): | |
"""Helper function to get speaker ID from voice name or ID""" | |
if hasattr(app_state, "voice_speaker_map") and voice in app_state.voice_speaker_map: | |
return app_state.voice_speaker_map[voice] | |
# Standard voices mapping | |
voice_to_speaker = {"alloy": 0, "echo": 1, "fable": 2, "onyx": 3, "nova": 4, "shimmer": 5} | |
if voice in voice_to_speaker: | |
return voice_to_speaker[voice] | |
# Try parsing as integer | |
try: | |
speaker_id = int(voice) | |
if 0 <= speaker_id < 6: | |
return speaker_id | |
except (ValueError, TypeError): | |
pass | |
# Check cloned voices if the voice cloner exists | |
if hasattr(app_state, "voice_cloner") and app_state.voice_cloner is not None: | |
# Check by ID | |
if voice in app_state.voice_cloner.cloned_voices: | |
return app_state.voice_cloner.cloned_voices[voice].speaker_id | |
# Check by name | |
for v_id, v_info in app_state.voice_cloner.cloned_voices.items(): | |
if v_info.name.lower() == voice.lower(): | |
return v_info.speaker_id | |
# Default to alloy | |
return 0 | |
async def generate_speech( | |
request: Request, | |
speech_request: SpeechRequest, | |
): | |
""" | |
Generate audio of text being spoken by a realistic voice. | |
This endpoint is compatible with the OpenAI TTS API. | |
For streaming responses, use `/v1/audio/speech/streaming` instead. | |
""" | |
# Check if model is available | |
if not hasattr(request.app.state, "generator") or request.app.state.generator is None: | |
raise HTTPException(status_code=503, detail="TTS model not available") | |
# Set default values | |
model = speech_request.model | |
voice = speech_request.voice | |
input_text = speech_request.input | |
response_format = speech_request.response_format | |
speed = speech_request.speed | |
temperature = speech_request.temperature | |
max_audio_length_ms = speech_request.max_audio_length_ms | |
# Log request details | |
logger.info(f"TTS request: text length={len(input_text)}, voice={voice}, format={response_format}") | |
try: | |
# Get speaker ID for the voice | |
speaker_id = get_speaker_id(request.app.state, voice) | |
if speaker_id is None: | |
raise HTTPException(status_code=400, detail=f"Voice '{voice}' not found") | |
# Check if this is a cloned voice | |
voice_info = None | |
cloned_voice_id = None | |
if hasattr(request.app.state, "get_voice_info"): | |
voice_info = request.app.state.get_voice_info(voice) | |
if voice_info and voice_info["type"] == "cloned": | |
cloned_voice_id = voice_info["voice_id"] | |
# Generate audio based on whether it's a standard or cloned voice | |
if cloned_voice_id is not None and hasattr(request.app.state, "voice_cloner"): | |
# Generate speech with cloned voice | |
logger.info(f"Generating speech with cloned voice ID: {cloned_voice_id}") | |
try: | |
voice_cloner = request.app.state.voice_cloner | |
audio = voice_cloner.generate_speech( | |
text=input_text, | |
voice_id=cloned_voice_id, | |
temperature=temperature, | |
topk=speech_request.topk or 30, | |
max_audio_length_ms=max_audio_length_ms | |
) | |
sample_rate = request.app.state.sample_rate | |
logger.info(f"Generated speech with cloned voice, length: {len(audio)/sample_rate:.2f}s") | |
except Exception as e: | |
logger.error(f"Error generating speech with cloned voice: {e}", exc_info=True) | |
raise HTTPException( | |
status_code=500, | |
detail=f"Failed to generate speech with cloned voice: {str(e)}" | |
) | |
else: | |
# Generate speech with standard voice | |
# Use voice context from memory if enabled | |
if hasattr(request.app.state, "voice_memory_enabled") and request.app.state.voice_memory_enabled: | |
from app.voice_memory import get_voice_context | |
context = get_voice_context(voice, torch.device(request.app.state.device)) | |
else: | |
context = [] | |
# Apply optional text enhancement for better voice consistency | |
enhanced_text = input_text | |
if hasattr(request.app.state, "prompt_templates"): | |
from app.prompt_engineering import format_text_for_voice | |
enhanced_text = format_text_for_voice(input_text, voice) | |
# Generate audio | |
audio = request.app.state.generator.generate( | |
text=enhanced_text, | |
speaker=speaker_id, | |
context=context, | |
temperature=temperature, | |
topk=speech_request.topk or 50, | |
max_audio_length_ms=max_audio_length_ms | |
) | |
sample_rate = request.app.state.sample_rate | |
# Process audio for better quality | |
if hasattr(request.app.state, "voice_enhancement_enabled") and request.app.state.voice_enhancement_enabled: | |
from app.voice_enhancement import process_generated_audio | |
audio = process_generated_audio( | |
audio=audio, | |
voice_name=voice, | |
sample_rate=sample_rate, | |
text=input_text | |
) | |
# Update voice memory if enabled | |
if hasattr(request.app.state, "voice_memory_enabled") and request.app.state.voice_memory_enabled: | |
from app.voice_memory import update_voice_memory | |
update_voice_memory(voice, audio, input_text) | |
# Handle speed adjustments if not 1.0 | |
if speed != 1.0 and speed > 0: | |
try: | |
# Adjust speed using torchaudio | |
effects = [ | |
["tempo", str(speed)] | |
] | |
audio_cpu = audio.cpu() | |
adjusted_audio, _ = torchaudio.sox_effects.apply_effects_tensor( | |
audio_cpu.unsqueeze(0), | |
sample_rate, | |
effects | |
) | |
audio = adjusted_audio.squeeze(0) | |
logger.info(f"Adjusted speech speed to {speed}x") | |
except Exception as e: | |
logger.warning(f"Failed to adjust speech speed: {e}") | |
# Format the audio according to the requested format | |
response_data, content_type = await format_audio( | |
audio, | |
response_format, | |
sample_rate, | |
request.app.state | |
) | |
# Create and return the response | |
return Response( | |
content=response_data, | |
media_type=content_type, | |
headers={"Content-Disposition": f"attachment; filename=speech.{response_format}"} | |
) | |
except Exception as e: | |
logger.error(f"Error in text_to_speech: {e}", exc_info=True) | |
raise HTTPException(status_code=500, detail=str(e)) | |
async def stream_speech(request: Request, speech_request: SpeechRequest): | |
"""Stream audio in real-time as it's being generated.""" | |
# Check if model is loaded | |
if not hasattr(request.app.state, "generator") or request.app.state.generator is None: | |
raise HTTPException(status_code=503, detail="Model not loaded") | |
# Get request parameters | |
input_text = speech_request.input | |
voice = speech_request.voice | |
response_format = speech_request.response_format | |
temperature = speech_request.temperature | |
logger.info(f"Real-time streaming speech from text ({len(input_text)} chars) with voice '{voice}'") | |
# Get speaker ID for the voice | |
speaker_id = get_speaker_id(request.app.state, voice) | |
if speaker_id is None: | |
raise HTTPException(status_code=400, detail=f"Voice '{voice}' not found") | |
# Split text into very small segments for incremental generation | |
text_segments = split_into_segments(input_text, max_chars=50) # Smaller segments for faster first response | |
logger.info(f"Split text into {len(text_segments)} segments") | |
# Create media type based on format | |
media_type = { | |
"mp3": "audio/mpeg", | |
"opus": "audio/opus", | |
"aac": "audio/aac", | |
"flac": "audio/flac", | |
"wav": "audio/wav", | |
}.get(response_format, "audio/mpeg") | |
# For streaming, WAV works best | |
streaming_format = "wav" | |
# Set up WAV header for streaming | |
sample_rate = request.app.state.sample_rate | |
async def generate_streaming_audio(): | |
# Get context for the voice | |
if hasattr(request.app.state, "voice_cloning_enabled") and request.app.state.voice_cloning_enabled: | |
voice_info = request.app.state.get_voice_info(voice) | |
if voice_info and voice_info["type"] == "cloned": | |
# Use cloned voice context | |
voice_cloner = request.app.state.voice_cloner | |
context = voice_cloner.get_voice_context(voice_info["voice_id"]) | |
else: | |
# Standard voice | |
from app.voice_enhancement import get_voice_segments | |
context = get_voice_segments(voice, request.app.state.device) | |
else: | |
# Standard voice | |
from app.voice_enhancement import get_voice_segments | |
context = get_voice_segments(voice, request.app.state.device) | |
# Send WAV header immediately | |
if streaming_format == "wav": | |
# Create a WAV header for 16-bit mono audio | |
header = bytes() | |
# RIFF header | |
header += b'RIFF' | |
header += b'\x00\x00\x00\x00' # Placeholder for file size | |
header += b'WAVE' | |
# Format chunk | |
header += b'fmt ' | |
header += (16).to_bytes(4, 'little') # Format chunk size | |
header += (1).to_bytes(2, 'little') # PCM format | |
header += (1).to_bytes(2, 'little') # Mono channel | |
header += (sample_rate).to_bytes(4, 'little') # Sample rate | |
header += (sample_rate * 2).to_bytes(4, 'little') # Byte rate | |
header += (2).to_bytes(2, 'little') # Block align | |
header += (16).to_bytes(2, 'little') # Bits per sample | |
# Data chunk | |
header += b'data' | |
header += b'\x00\x00\x00\x00' # Placeholder for data size | |
yield header | |
# Process each segment and stream immediately | |
for i, segment_text in enumerate(text_segments): | |
try: | |
logger.info(f"Generating segment {i+1}/{len(text_segments)}") | |
# For cloned voices, use the voice cloner | |
if hasattr(request.app.state, "voice_cloning_enabled") and request.app.state.voice_cloning_enabled: | |
voice_info = request.app.state.get_voice_info(voice) | |
if voice_info and voice_info["type"] == "cloned": | |
# Use cloned voice | |
voice_cloner = request.app.state.voice_cloner | |
segment_audio = await asyncio.to_thread( | |
voice_cloner.generate_speech, | |
segment_text, | |
voice_info["voice_id"], | |
temperature=temperature, | |
topk=30, | |
max_audio_length_ms=2000 # Keep it very short for fast generation | |
) | |
else: | |
# Use standard voice with generator | |
segment_audio = await asyncio.to_thread( | |
request.app.state.generator.generate, | |
segment_text, | |
speaker_id, | |
context, | |
max_audio_length_ms=2000, # Short for quicker generation | |
temperature=temperature | |
) | |
else: | |
# Use standard voice with generator | |
segment_audio = await asyncio.to_thread( | |
request.app.state.generator.generate, | |
segment_text, | |
speaker_id, | |
context, | |
max_audio_length_ms=2000, # Short for quicker generation | |
temperature=temperature | |
) | |
# Skip empty or problematic audio | |
if segment_audio is None or segment_audio.numel() == 0: | |
logger.warning(f"Empty audio for segment {i+1}") | |
continue | |
# Convert to bytes and stream immediately | |
buf = io.BytesIO() | |
audio_to_save = segment_audio.unsqueeze(0) if len(segment_audio.shape) == 1 else segment_audio | |
torchaudio.save(buf, audio_to_save.cpu(), sample_rate, format=streaming_format) | |
buf.seek(0) | |
# For WAV format, skip the header for all segments after the first | |
if streaming_format == "wav" and i > 0: | |
buf.seek(44) # Skip WAV header | |
segment_bytes = buf.read() | |
yield segment_bytes | |
# Update context with this segment for next generation | |
context = [ | |
Segment( | |
text=segment_text, | |
speaker=speaker_id, | |
audio=segment_audio | |
) | |
] | |
except Exception as e: | |
logger.error(f"Error generating segment {i+1}: {e}") | |
# Continue to next segment | |
# Return the streaming response | |
return StreamingResponse( | |
generate_streaming_audio(), | |
media_type=media_type, | |
headers={ | |
"X-Accel-Buffering": "no", # Prevent buffering in nginx | |
"Cache-Control": "no-cache, no-store, must-revalidate", | |
"Connection": "keep-alive", | |
"Transfer-Encoding": "chunked" | |
} | |
) | |
async def openai_stream_speech( | |
request: Request, | |
speech_request: SpeechRequest, | |
): | |
""" | |
Stream audio in OpenAI-compatible streaming format. | |
This endpoint is compatible with the OpenAI streaming TTS API. | |
""" | |
# Use the same logic as the stream_speech endpoint but with a different name | |
# to maintain the OpenAI API naming convention | |
return await stream_speech(request, speech_request) | |
async def format_audio(audio, response_format, sample_rate, app_state): | |
""" | |
Format audio according to requested format. | |
Args: | |
audio: Audio tensor from TTS generation | |
response_format: Format as string or enum ('mp3', 'opus', 'aac', 'flac', 'wav') | |
sample_rate: Sample rate of the audio | |
app_state: FastAPI app state with config and cache settings | |
Returns: | |
Tuple of (response_data, content_type) | |
""" | |
import io | |
import torch | |
import torchaudio | |
import tempfile | |
import os | |
import hashlib | |
import time | |
# Handle enum or string for response_format | |
if hasattr(response_format, 'value'): | |
response_format = response_format.value | |
# Normalize response_format to lowercase | |
response_format = str(response_format).lower() | |
# Map formats to content types | |
format_to_content_type = { | |
'mp3': 'audio/mpeg', | |
'opus': 'audio/opus', | |
'aac': 'audio/aac', | |
'flac': 'audio/flac', | |
'wav': 'audio/wav' | |
} | |
# Ensure response format is supported | |
if response_format not in format_to_content_type: | |
logger.warning(f"Unsupported format: {response_format}, defaulting to mp3") | |
response_format = 'mp3' | |
# Generate a cache key based on audio content and format | |
cache_enabled = getattr(app_state, "audio_cache_enabled", False) | |
cache_key = None | |
if cache_enabled: | |
# Generate a hash of the audio tensor for caching | |
audio_hash = hashlib.md5(audio.cpu().numpy().tobytes()).hexdigest() | |
cache_key = f"{audio_hash}_{response_format}" | |
cache_dir = getattr(app_state, "audio_cache_dir", "/app/audio_cache") | |
os.makedirs(cache_dir, exist_ok=True) | |
cache_path = os.path.join(cache_dir, f"{cache_key}") | |
# Check if we have a cache hit | |
if os.path.exists(cache_path): | |
try: | |
with open(cache_path, "rb") as f: | |
cached_data = f.read() | |
logger.info(f"Cache hit for {response_format} audio") | |
return cached_data, format_to_content_type[response_format] | |
except Exception as e: | |
logger.warning(f"Error reading from cache: {e}") | |
# Process audio to the required format | |
start_time = time.time() | |
# Move audio to CPU before saving | |
audio_cpu = audio.cpu() | |
# Use a temporary file for format conversion | |
with tempfile.NamedTemporaryFile(suffix=f".{response_format}", delete=False) as temp_file: | |
temp_path = temp_file.name | |
try: | |
if response_format == 'wav': | |
# Direct save for WAV | |
torchaudio.save(temp_path, audio_cpu.unsqueeze(0), sample_rate) | |
else: | |
# For other formats, first save as WAV then convert | |
wav_path = f"{temp_path}.wav" | |
torchaudio.save(wav_path, audio_cpu.unsqueeze(0), sample_rate) | |
# Use ffmpeg via torchaudio for conversion | |
if hasattr(torchaudio.backend, 'sox_io_backend'): # New torchaudio structure | |
if response_format == 'mp3': | |
# For MP3, use higher quality | |
sox_effects = torchaudio.sox_effects.SoxEffectsChain() | |
sox_effects.set_input_file(wav_path) | |
sox_effects.append_effect_to_chain(["rate", f"{sample_rate}"]) | |
# Higher bitrate for better quality | |
sox_effects.append_effect_to_chain(["gain", "-n"]) # Normalize | |
out, _ = sox_effects.sox_build_flow_effects() | |
torchaudio.save(temp_path, out, sample_rate, format="mp3", compression=128) | |
elif response_format == 'opus': | |
# Use ffmpeg for opus through a system call | |
import subprocess | |
subprocess.run([ | |
"ffmpeg", "-i", wav_path, "-c:a", "libopus", | |
"-b:a", "64k", "-vbr", "on", temp_path, | |
"-y", "-loglevel", "error" | |
], check=True) | |
elif response_format == 'aac': | |
# Use ffmpeg for AAC through a system call | |
import subprocess | |
subprocess.run([ | |
"ffmpeg", "-i", wav_path, "-c:a", "aac", | |
"-b:a", "128k", temp_path, | |
"-y", "-loglevel", "error" | |
], check=True) | |
elif response_format == 'flac': | |
torchaudio.save(temp_path, audio_cpu.unsqueeze(0), sample_rate, format="flac") | |
else: | |
# Fallback using external command | |
import subprocess | |
if response_format == 'mp3': | |
subprocess.run([ | |
"ffmpeg", "-i", wav_path, "-codec:a", "libmp3lame", | |
"-qscale:a", "2", temp_path, | |
"-y", "-loglevel", "error" | |
], check=True) | |
elif response_format == 'opus': | |
subprocess.run([ | |
"ffmpeg", "-i", wav_path, "-c:a", "libopus", | |
"-b:a", "64k", "-vbr", "on", temp_path, | |
"-y", "-loglevel", "error" | |
], check=True) | |
elif response_format == 'aac': | |
subprocess.run([ | |
"ffmpeg", "-i", wav_path, "-c:a", "aac", | |
"-b:a", "128k", temp_path, | |
"-y", "-loglevel", "error" | |
], check=True) | |
elif response_format == 'flac': | |
subprocess.run([ | |
"ffmpeg", "-i", wav_path, "-c:a", "flac", temp_path, | |
"-y", "-loglevel", "error" | |
], check=True) | |
# Clean up the temporary WAV file | |
try: | |
os.unlink(wav_path) | |
except: | |
pass | |
# Read the processed audio file | |
with open(temp_path, "rb") as f: | |
response_data = f.read() | |
# Store in cache if enabled | |
if cache_enabled and cache_key: | |
try: | |
cache_path = os.path.join(getattr(app_state, "audio_cache_dir", "/app/audio_cache"), f"{cache_key}") | |
with open(cache_path, "wb") as f: | |
f.write(response_data) | |
logger.debug(f"Cached {response_format} audio with key: {cache_key}") | |
except Exception as e: | |
logger.warning(f"Error writing to cache: {e}") | |
# Log processing time | |
processing_time = time.time() - start_time | |
logger.info(f"Processed audio to {response_format} in {processing_time:.3f}s") | |
return response_data, format_to_content_type[response_format] | |
except Exception as e: | |
logger.error(f"Error converting audio to {response_format}: {e}") | |
# Fallback to WAV if conversion fails | |
try: | |
wav_path = f"{temp_path}.wav" | |
torchaudio.save(wav_path, audio_cpu.unsqueeze(0), sample_rate) | |
with open(wav_path, "rb") as f: | |
response_data = f.read() | |
os.unlink(wav_path) | |
return response_data, "audio/wav" | |
except Exception as fallback_error: | |
logger.error(f"Fallback to WAV also failed: {fallback_error}") | |
raise RuntimeError(f"Failed to generate audio in any format: {str(e)}") | |
finally: | |
# Clean up the temporary file | |
try: | |
os.unlink(temp_path) | |
except: | |
pass | |
async def conversation_to_speech( | |
request: Request, | |
text: str = Body(..., description="Text to convert to speech"), | |
speaker_id: int = Body(0, description="Speaker ID"), | |
context: List[Dict] = Body([], description="Context segments with speaker, text, and audio path"), | |
): | |
""" | |
Custom endpoint for conversational TTS using CSM-1B. | |
This is not part of the OpenAI API but provides the unique conversational | |
capability of the CSM model. | |
""" | |
# Get generator from app state | |
generator = request.app.state.generator | |
# Validate model availability | |
if generator is None: | |
raise HTTPException(status_code=503, detail="Model not loaded") | |
try: | |
segments = [] | |
# Process context if provided | |
for ctx in context: | |
if 'speaker' not in ctx or 'text' not in ctx or 'audio' not in ctx: | |
continue | |
# Audio should be base64-encoded | |
audio_data = base64.b64decode(ctx['audio']) | |
audio_file = io.BytesIO(audio_data) | |
# Save to temporary file for torchaudio | |
with tempfile.NamedTemporaryFile(suffix=".wav", delete=False) as temp: | |
temp.write(audio_file.read()) | |
temp_path = temp.name | |
# Load audio | |
audio_tensor, sample_rate = torchaudio.load(temp_path) | |
audio_tensor = torchaudio.functional.resample( | |
audio_tensor.squeeze(0), | |
orig_freq=sample_rate, | |
new_freq=generator.sample_rate | |
) | |
# Clean up | |
os.unlink(temp_path) | |
# Create segment | |
segments.append( | |
Segment( | |
speaker=ctx['speaker'], | |
text=ctx['text'], | |
audio=audio_tensor | |
) | |
) | |
logger.info(f"Conversation request: '{text}' with {len(segments)} context segments") | |
# Format the text for better voice consistency | |
from app.prompt_engineering import format_text_for_voice | |
# Determine voice name from speaker_id | |
voice_names = ["alloy", "echo", "fable", "onyx", "nova", "shimmer"] | |
voice_name = voice_names[speaker_id] if 0 <= speaker_id < len(voice_names) else "alloy" | |
formatted_text = format_text_for_voice(text, voice_name) | |
# Generate audio with context | |
audio = generator.generate( | |
text=formatted_text, | |
speaker=speaker_id, | |
context=segments, | |
max_audio_length_ms=20000, # 20 seconds | |
temperature=0.7, # Lower temperature for more stable output | |
topk=40, | |
) | |
# Process audio for better quality | |
from app.voice_enhancement import process_generated_audio | |
processed_audio = process_generated_audio( | |
audio, | |
voice_name, | |
generator.sample_rate, | |
text | |
) | |
# Save to temporary file | |
with tempfile.NamedTemporaryFile(suffix=".wav", delete=False) as temp: | |
temp_path = temp.name | |
# Save audio | |
torchaudio.save(temp_path, processed_audio.unsqueeze(0).cpu(), generator.sample_rate) | |
# Return audio file | |
def iterfile(): | |
with open(temp_path, 'rb') as f: | |
yield from f | |
# Clean up | |
if os.path.exists(temp_path): | |
os.unlink(temp_path) | |
logger.info(f"Generated conversation response, duration: {processed_audio.shape[0]/generator.sample_rate:.2f}s") | |
return StreamingResponse( | |
iterfile(), | |
media_type="audio/wav", | |
headers={'Content-Disposition': 'attachment; filename="speech.wav"'} | |
) | |
except Exception as e: | |
import traceback | |
error_trace = traceback.format_exc() | |
logger.error(f"Conversation speech generation failed: {str(e)}\n{error_trace}") | |
raise HTTPException(status_code=500, detail=f"Conversation speech generation failed: {str(e)}") | |
async def list_voices(request: Request): | |
""" | |
List available voices in a format compatible with OpenAI and OpenWebUI. | |
""" | |
# Use app state's get_all_voices function if available | |
if hasattr(request.app.state, "get_all_voices"): | |
voices = request.app.state.get_all_voices() | |
logger.info(f"Listing {len(voices)} voices") | |
return {"voices": voices} | |
# Fallback to standard voices if necessary | |
standard_voices = [ | |
{"voice_id": "alloy", "name": "Alloy"}, | |
{"voice_id": "echo", "name": "Echo"}, | |
{"voice_id": "fable", "name": "Fable"}, | |
{"voice_id": "onyx", "name": "Onyx"}, | |
{"voice_id": "nova", "name": "Nova"}, | |
{"voice_id": "shimmer", "name": "Shimmer"} | |
] | |
# Add cloned voices if available | |
if hasattr(request.app.state, "voice_cloner") and request.app.state.voice_cloner is not None: | |
cloned_voices = request.app.state.voice_cloner.list_voices() | |
for voice in cloned_voices: | |
standard_voices.append({ | |
"voice_id": voice.id, # This has to be specifically voice_id | |
"name": voice.name # This has to be specifically name | |
}) | |
logger.info(f"Listing {len(standard_voices)} voices") | |
return {"voices": standard_voices} | |
# Add OpenAI-compatible models list endpoint | |
async def list_models(): | |
""" | |
OpenAI compatible endpoint that returns a list of available audio models. | |
""" | |
models = [ | |
{ | |
"id": "csm-1b", | |
"name": "CSM-1B", | |
"description": "Conversational Speech Model 1B from Sesame", | |
"created": 1716019200, # March 13, 2025 (from the example) | |
"object": "audio", | |
"owned_by": "sesame", | |
"capabilities": { | |
"tts": True, | |
"voice_generation": True, | |
"voice_cloning": hasattr(router.app, "voice_cloner"), | |
"streaming": True | |
}, | |
"max_input_length": 4096, | |
"price": {"text-to-speech": 0.00} | |
}, | |
{ | |
"id": "tts-1", | |
"name": "CSM-1B (Compatibility Mode)", | |
"description": "CSM-1B with OpenAI TTS-1 compatibility", | |
"created": 1716019200, | |
"object": "audio", | |
"owned_by": "sesame", | |
"capabilities": { | |
"tts": True, | |
"voice_generation": True, | |
"streaming": True | |
}, | |
"max_input_length": 4096, | |
"price": {"text-to-speech": 0.00} | |
}, | |
{ | |
"id": "tts-1-hd", | |
"name": "CSM-1B (HD Mode)", | |
"description": "CSM-1B with higher quality settings", | |
"created": 1716019200, | |
"object": "audio", | |
"owned_by": "sesame", | |
"capabilities": { | |
"tts": True, | |
"voice_generation": True, | |
"streaming": True | |
}, | |
"max_input_length": 4096, | |
"price": {"text-to-speech": 0.00} | |
} | |
] | |
return {"data": models, "object": "list"} | |
# Response format options endpoint | |
async def list_response_formats(): | |
"""List available response formats for speech synthesis.""" | |
formats = [ | |
{"name": "mp3", "content_type": "audio/mpeg"}, | |
{"name": "opus", "content_type": "audio/opus"}, | |
{"name": "aac", "content_type": "audio/aac"}, | |
{"name": "flac", "content_type": "audio/flac"}, | |
{"name": "wav", "content_type": "audio/wav"} | |
] | |
return {"response_formats": formats} | |
# Streaming format options endpoint | |
async def list_stream_formats(): | |
"""List available streaming formats for TTS.""" | |
return { | |
"stream_formats": [ | |
{ | |
"format": "mp3", | |
"content_type": "audio/mpeg", | |
"description": "MP3 audio format (streaming)" | |
}, | |
{ | |
"format": "opus", | |
"content_type": "audio/opus", | |
"description": "Opus audio format (streaming)" | |
}, | |
{ | |
"format": "aac", | |
"content_type": "audio/aac", | |
"description": "AAC audio format (streaming)" | |
}, | |
{ | |
"format": "flac", | |
"content_type": "audio/flac", | |
"description": "FLAC audio format (streaming)" | |
}, | |
{ | |
"format": "wav", | |
"content_type": "audio/wav", | |
"description": "WAV audio format (streaming)" | |
} | |
] | |
} | |
# Simple test endpoint | |
async def test_endpoint(): | |
"""Simple test endpoint that returns a successful response.""" | |
return {"status": "ok", "message": "API is working"} | |
# Debug endpoint | |
async def debug_info(request: Request): | |
"""Get debug information about the API.""" | |
generator = request.app.state.generator | |
# Basic info | |
debug_info = { | |
"model_loaded": generator is not None, | |
"device": generator.device if generator is not None else None, | |
"sample_rate": generator.sample_rate if generator is not None else None, | |
} | |
# Add voice enhancement info if available | |
try: | |
from app.voice_enhancement import VOICE_PROFILES | |
voice_info = {} | |
for name, profile in VOICE_PROFILES.items(): | |
voice_info[name] = { | |
"pitch_range": f"{profile.pitch_range[0]}-{profile.pitch_range[1]}Hz", | |
"timbre": profile.timbre, | |
"ref_segments": len(profile.reference_segments), | |
} | |
debug_info["voice_profiles"] = voice_info | |
except ImportError: | |
debug_info["voice_profiles"] = "Not available" | |
# Add voice cloning info if available | |
if hasattr(request.app.state, "voice_cloner"): | |
voice_cloner = request.app.state.voice_cloner | |
debug_info["voice_cloning"] = { | |
"enabled": True, | |
"cloned_voices_count": len(voice_cloner.list_voices()), | |
"cloned_voices": [v.name for v in voice_cloner.list_voices()] | |
} | |
else: | |
debug_info["voice_cloning"] = {"enabled": False} | |
# Add streaming info | |
debug_info["streaming"] = {"enabled": True} | |
# Add memory usage info for CUDA | |
if torch.cuda.is_available(): | |
debug_info["cuda"] = { | |
"allocated_memory_gb": torch.cuda.memory_allocated() / 1e9, | |
"reserved_memory_gb": torch.cuda.memory_reserved() / 1e9, | |
"max_memory_gb": torch.cuda.get_device_properties(0).total_memory / 1e9, | |
} | |
return debug_info | |
async def get_voice_storage_info(request: Request): | |
"""Get information about voice storage usage and status.""" | |
from app.utils.voice_manager import get_voice_storage_info | |
return get_voice_storage_info() | |
async def create_voice_backup(request: Request): | |
"""Create a backup of all voice data.""" | |
from app.utils.voice_manager import backup_voice_data | |
backup_path = backup_voice_data() | |
return {"status": "success", "backup_path": backup_path} | |
async def reset_voices(request: Request): | |
"""Reset voices to their default state.""" | |
from app.utils.voice_manager import restore_default_voices | |
backup_path = restore_default_voices() | |
return {"status": "success", "backup_path": backup_path, "message": "Voices reset to default state"} | |
async def verify_references(request: Request): | |
"""Check if voice references are complete and valid.""" | |
from app.utils.voice_manager import verify_voice_references | |
return verify_voice_references() | |
# Voice diagnostics endpoint | |
async def voice_diagnostics(): | |
"""Get diagnostic information about voice references.""" | |
try: | |
from app.voice_enhancement import VOICE_PROFILES | |
diagnostics = {} | |
for name, profile in VOICE_PROFILES.items(): | |
ref_info = [] | |
for i, ref in enumerate(profile.reference_segments): | |
if ref is not None: | |
duration = ref.shape[0] / 24000 # Assume 24kHz | |
ref_info.append({ | |
"index": i, | |
"duration_seconds": f"{duration:.2f}", | |
"samples": ref.shape[0], | |
"min": float(ref.min()), | |
"max": float(ref.max()), | |
"rms": float(torch.sqrt(torch.mean(ref ** 2))), | |
}) | |
diagnostics[name] = { | |
"speaker_id": profile.speaker_id, | |
"pitch_range": f"{profile.pitch_range[0]}-{profile.pitch_range[1]}Hz", | |
"references": ref_info, | |
"reference_count": len(ref_info), | |
} | |
return {"diagnostics": diagnostics} | |
except ImportError: | |
return {"error": "Voice enhancement module not available"} | |
# Specialized debugging endpoint for speech generation | |
async def debug_speech( | |
request: Request, | |
text: str = Body(..., embed=True), | |
voice: str = Body("alloy", embed=True), | |
use_enhancement: bool = Body(True, embed=True) | |
): | |
"""Debug endpoint for speech generation with enhancement options.""" | |
generator = request.app.state.generator | |
if generator is None: | |
return {"error": "Model not loaded"} | |
try: | |
# Convert voice name to speaker ID | |
voice_map = { | |
"alloy": 0, | |
"echo": 1, | |
"fable": 2, | |
"onyx": 3, | |
"nova": 4, | |
"shimmer": 5 | |
} | |
speaker = voice_map.get(voice, 0) | |
# Format text if using enhancement | |
if use_enhancement: | |
from app.prompt_engineering import format_text_for_voice | |
formatted_text = format_text_for_voice(text, voice) | |
logger.info(f"Using formatted text: {formatted_text}") | |
else: | |
formatted_text = text | |
# Get context if using enhancement | |
if use_enhancement: | |
from app.voice_enhancement import get_voice_segments | |
context = get_voice_segments(voice, generator.device) | |
logger.info(f"Using {len(context)} context segments") | |
else: | |
context = [] | |
# Generate audio | |
start_time = time.time() | |
audio = generator.generate( | |
text=formatted_text, | |
speaker=speaker, | |
context=context, | |
max_audio_length_ms=10000, # 10 seconds | |
temperature=0.7 if use_enhancement else 0.9, | |
topk=40 if use_enhancement else 50, | |
) | |
generation_time = time.time() - start_time | |
# Process audio if using enhancement | |
if use_enhancement: | |
from app.voice_enhancement import process_generated_audio | |
start_time = time.time() | |
processed_audio = process_generated_audio(audio, voice, generator.sample_rate, text) | |
processing_time = time.time() - start_time | |
else: | |
processed_audio = audio | |
processing_time = 0 | |
# Save to temporary WAV file | |
temp_path = f"/tmp/debug_speech_{voice}_{int(time.time())}.wav" | |
torchaudio.save(temp_path, processed_audio.unsqueeze(0).cpu(), generator.sample_rate) | |
# Also save original if enhanced | |
if use_enhancement: | |
orig_path = f"/tmp/debug_speech_{voice}_original_{int(time.time())}.wav" | |
torchaudio.save(orig_path, audio.unsqueeze(0).cpu(), generator.sample_rate) | |
else: | |
orig_path = temp_path | |
# Calculate audio metrics | |
duration = processed_audio.shape[0] / generator.sample_rate | |
rms = float(torch.sqrt(torch.mean(processed_audio ** 2))) | |
peak = float(processed_audio.abs().max()) | |
return { | |
"status": "success", | |
"message": f"Audio generated successfully and saved to {temp_path}", | |
"audio": { | |
"duration_seconds": f"{duration:.2f}", | |
"samples": processed_audio.shape[0], | |
"sample_rate": generator.sample_rate, | |
"rms_level": f"{rms:.3f}", | |
"peak_level": f"{peak:.3f}", | |
}, | |
"processing": { | |
"enhancement_used": use_enhancement, | |
"generation_time_seconds": f"{generation_time:.3f}", | |
"processing_time_seconds": f"{processing_time:.3f}", | |
"original_path": orig_path, | |
"processed_path": temp_path, | |
} | |
} | |
except Exception as e: | |
import traceback | |
error_trace = traceback.format_exc() | |
logger.error(f"Debug speech generation failed: {e}\n{error_trace}") | |
return { | |
"status": "error", | |
"message": str(e), | |
"traceback": error_trace | |
} | |
async def transcribe_audio( | |
request: Request, | |
audio: UploadFile = File(...), | |
language: Optional[str] = Query(None, description="Language code (e.g., 'en', 'fr', 'de')"), | |
align_text: bool = Query(False, description="Whether to align text with timestamps"), | |
compute_type: str = Query("float16", description="Compute type for model inference (float16, int8, float32)"), | |
): | |
""" | |
Transcribe spoken audio to text using WhisperX (faster and more accurate). | |
Upload audio as a file in any common format (mp3, wav, etc.). | |
**Parameters:** | |
- `audio`: Audio file to transcribe | |
- `language`: Optional language code (auto-detected if not provided) | |
- `align_text`: Whether to include word-level timestamps | |
- `compute_type`: Compute type for model inference (float16, int8, float32) | |
**Response:** | |
```json | |
{ | |
"text": "Transcribed text", | |
"segments": [ | |
{ | |
"start": 0.0, | |
"end": 2.5, | |
"text": "Segment text" | |
} | |
], | |
"word_timestamps": [] // If align_text is true | |
} | |
``` | |
""" | |
global whisperx_model | |
# Create temp directory to store uploaded file | |
with tempfile.TemporaryDirectory() as temp_dir: | |
temp_path = os.path.join(temp_dir, f"audio_upload{os.path.splitext(audio.filename)[1]}") | |
# Save uploaded file to temp directory | |
try: | |
content = await audio.read() | |
with open(temp_path, "wb") as f: | |
f.write(content) | |
logger.info(f"Saved uploaded audio to {temp_path}") | |
# Use lock to ensure model loading is thread-safe | |
async with whisperx_model_lock: | |
# Load WhisperX model if not already loaded | |
if whisperx_model is None: | |
logger.info("Loading WhisperX model (one-time initialization)") | |
device = "cuda" if torch.cuda.is_available() else "cpu" | |
# Use medium model for better accuracy, but small can be used for faster processing | |
whisperx_model = whisperx.load_model("medium", device, compute_type=compute_type, asr_options={"beam_size": 5}) | |
logger.info(f"WhisperX model loaded on {device} with compute_type={compute_type}") | |
# Start processing timer | |
start_time = time.time() | |
# Specify device for batch processing | |
device = "cuda" if torch.cuda.is_available() else "cpu" | |
# Transcribe with WhisperX (much faster than standard whisper) | |
logger.info(f"Transcribing audio with WhisperX on {device}") | |
# Process audio file - much faster than standard whisper | |
# and can process batches concurrently on GPU | |
result = whisperx_model.transcribe( | |
temp_path, | |
language=language, | |
batch_size=16 if device == "cuda" else 1 # Larger batch size for GPU | |
) | |
# Align word timestamps if requested | |
if align_text and result["segments"]: | |
try: | |
# Load alignment model | |
logger.info("Aligning text with timestamps") | |
alignment_model, metadata = whisperx.load_align_model( | |
language_code=result["language"] if language is None else language, | |
device=device | |
) | |
# Align | |
result = whisperx.align( | |
result["segments"], | |
alignment_model, | |
metadata, | |
temp_path, | |
device, | |
return_char_alignments=False | |
) | |
except Exception as e: | |
logger.warning(f"Word alignment failed: {e}") | |
# Continue without alignment if it fails | |
# Calculate processing time | |
processing_time = time.time() - start_time | |
# Log results | |
logger.info(f"Successfully transcribed audio in {processing_time:.2f}s: {result['text'][:50]}...") | |
# Return results | |
response = { | |
"text": result["text"], | |
"segments": result["segments"], | |
"language": result.get("language", language), | |
"processing_time": processing_time | |
} | |
# Add word timestamps if available | |
if align_text and "word_segments" in result: | |
response["word_timestamps"] = result["word_segments"] | |
return response | |
except Exception as e: | |
logger.error(f"Transcription failed: {e}", exc_info=True) | |
raise HTTPException( | |
status_code=500, | |
detail=f"Failed to transcribe audio: {str(e)}" | |
) |