Spaces:
Running
Running
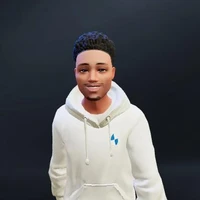
Refactor websocket_conversation function to simplify access to app state: remove request parameter and directly use websocket.app for model availability checks and audio processing tasks.
a536271
"""Real-time audio conversation with WebSockets. | |
This module provides WebSocket endpoints for real-time audio conversation | |
using the CSM-1B model and WhisperX for transcription. | |
""" | |
import os | |
import io | |
import base64 | |
import json | |
import time | |
import asyncio | |
import logging | |
import tempfile | |
from enum import Enum | |
from typing import Dict, List, Optional, Any, Union | |
import numpy as np | |
import torch | |
import torchaudio | |
from pydub import AudioSegment | |
import whisperx | |
from fastapi import APIRouter, WebSocket, WebSocketDisconnect, HTTPException, Request | |
from fastapi.responses import JSONResponse | |
# Set up logging | |
logger = logging.getLogger(__name__) | |
router = APIRouter(prefix="/realtime", tags=["Real-time Conversation"]) | |
# Audio processing constants | |
SAMPLE_RATE = 16000 # Sample rate for audio processing | |
CHUNK_SIZE = 4096 # Chunk size for audio processing | |
MAX_AUDIO_DURATION = 10 # Maximum audio duration in seconds | |
SILENCE_THRESHOLD = 400 # Threshold for detecting silence (RMS) | |
MIN_SILENCE_DURATION = 0.5 # Minimum silence duration to consider a pause | |
# WebSocket message types | |
class MessageType(str, Enum): | |
AUDIO_CHUNK = "audio_chunk" | |
TRANSCRIPT = "transcript" | |
RESPONSE = "response" | |
START_SPEAKING = "start_speaking" | |
STOP_SPEAKING = "stop_speaking" | |
ERROR = "error" | |
STATUS = "status" | |
# WhisperX model cache for performance | |
_whisperx_model = None | |
_whisperx_model_lock = asyncio.Lock() | |
# Connection manager for websockets | |
class ConnectionManager: | |
def __init__(self): | |
self.active_connections: Dict[str, WebSocket] = {} | |
self.conversation_contexts: Dict[str, List] = {} | |
self.voice_preferences: Dict[str, int] = {} # Store voice preferences by client_id | |
async def connect(self, websocket: WebSocket, client_id: str): | |
"""Connect a client to the WebSocket""" | |
await websocket.accept() | |
self.active_connections[client_id] = websocket | |
self.conversation_contexts[client_id] = [] | |
self.voice_preferences[client_id] = 1 # Default to echo voice | |
logger.info(f"Client {client_id} connected, active connections: {len(self.active_connections)}") | |
def disconnect(self, client_id: str): | |
"""Disconnect a client from the WebSocket""" | |
if client_id in self.active_connections: | |
del self.active_connections[client_id] | |
if client_id in self.conversation_contexts: | |
del self.conversation_contexts[client_id] | |
if client_id in self.voice_preferences: | |
del self.voice_preferences[client_id] | |
logger.info(f"Client {client_id} disconnected, active connections: {len(self.active_connections)}") | |
def set_voice_preference(self, client_id: str, speaker_id: int): | |
"""Set voice preference for a client""" | |
self.voice_preferences[client_id] = speaker_id | |
def get_voice_preference(self, client_id: str) -> int: | |
"""Get voice preference for a client""" | |
return self.voice_preferences.get(client_id, 1) # Default to echo (speaker_id=1) | |
async def send_message(self, client_id: str, message_type: MessageType, data: Any): | |
"""Send a message to a client""" | |
if client_id in self.active_connections: | |
message = { | |
"type": message_type, | |
"data": data, | |
"timestamp": time.time() | |
} | |
await self.active_connections[client_id].send_json(message) | |
def add_to_context(self, client_id: str, speaker: int, text: str, audio: Union[torch.Tensor, bytes]): | |
"""Add a message to the conversation context""" | |
if client_id in self.conversation_contexts: | |
# Convert audio tensor to base64 if needed | |
if isinstance(audio, torch.Tensor): | |
audio_bytes = convert_tensor_to_wav_bytes(audio) | |
audio_base64 = base64.b64encode(audio_bytes).decode('utf-8') | |
elif isinstance(audio, bytes): | |
audio_base64 = base64.b64encode(audio).decode('utf-8') | |
else: | |
raise ValueError(f"Unsupported audio type: {type(audio)}") | |
# Add to context, limiting size to last 5 exchanges | |
self.conversation_contexts[client_id].append({ | |
"speaker": speaker, | |
"text": text, | |
"audio": audio_base64 | |
}) | |
# Limit context size (keep last 5 exchanges to prevent context growing too large) | |
if len(self.conversation_contexts[client_id]) > 5: | |
self.conversation_contexts[client_id] = self.conversation_contexts[client_id][-5:] | |
def get_context(self, client_id: str) -> List[Dict]: | |
"""Get the conversation context for a client""" | |
return self.conversation_contexts.get(client_id, []) | |
# Initialize connection manager | |
manager = ConnectionManager() | |
async def load_whisperx_model(compute_type="float16"): | |
"""Load WhisperX model if not already loaded""" | |
global _whisperx_model | |
# Use lock to ensure model loading is thread-safe | |
async with _whisperx_model_lock: | |
# Load WhisperX model if not already loaded | |
if _whisperx_model is None: | |
logger.info("Loading WhisperX model for real-time transcription") | |
device = "cuda" if torch.cuda.is_available() else "cpu" | |
# Use small model for lower latency | |
_whisperx_model = whisperx.load_model( | |
"small", # Small model for faster processing in real-time | |
device, | |
compute_type=compute_type, | |
asr_options={"beam_size": 5, "vad_onset": 0.5, "vad_offset": 0.5} | |
) | |
logger.info(f"WhisperX model loaded on {device} with compute_type={compute_type}") | |
return _whisperx_model | |
def convert_tensor_to_wav_bytes(audio_tensor: torch.Tensor) -> bytes: | |
"""Convert audio tensor to WAV bytes""" | |
buf = io.BytesIO() | |
if len(audio_tensor.shape) == 1: | |
audio_tensor = audio_tensor.unsqueeze(0) | |
torchaudio.save(buf, audio_tensor.cpu(), SAMPLE_RATE, format="wav") | |
buf.seek(0) | |
return buf.read() | |
def convert_audio_data(audio_data: bytes) -> torch.Tensor: | |
"""Convert audio data to tensor""" | |
with tempfile.NamedTemporaryFile(suffix=".wav", delete=True) as temp: | |
temp.write(audio_data) | |
temp.flush() | |
# Load audio | |
try: | |
# First try with torchaudio | |
waveform, sample_rate = torchaudio.load(temp.name) | |
# Convert to mono if needed | |
if waveform.shape[0] > 1: | |
waveform = torch.mean(waveform, dim=0, keepdim=True) | |
# Resample if needed | |
if sample_rate != SAMPLE_RATE: | |
waveform = torchaudio.functional.resample( | |
waveform, orig_freq=sample_rate, new_freq=SAMPLE_RATE | |
) | |
return waveform.squeeze(0) | |
except: | |
# Fallback to pydub if torchaudio fails | |
audio = AudioSegment.from_file(temp.name) | |
# Convert to mono if needed | |
if audio.channels > 1: | |
audio = audio.set_channels(1) | |
# Resample if needed | |
if audio.frame_rate != SAMPLE_RATE: | |
audio = audio.set_frame_rate(SAMPLE_RATE) | |
# Convert to numpy array | |
samples = np.array(audio.get_array_of_samples(), dtype=np.float32) / 32768.0 | |
# Convert to tensor | |
waveform = torch.tensor(samples, dtype=torch.float32) | |
return waveform | |
async def transcribe_audio(audio_data: bytes, language: Optional[str] = None) -> Dict: | |
"""Transcribe audio using WhisperX""" | |
# Load WhisperX model | |
model = await load_whisperx_model() | |
# Save audio to temporary file | |
with tempfile.NamedTemporaryFile(suffix=".wav", delete=True) as temp: | |
temp.write(audio_data) | |
temp.flush() | |
# Transcribe with WhisperX | |
device = "cuda" if torch.cuda.is_available() else "cpu" | |
result = model.transcribe( | |
temp.name, | |
language=language, | |
batch_size=16 if device == "cuda" else 1 | |
) | |
return result | |
async def generate_response(app, text: str, speaker_id: int, context: List[Dict]) -> torch.Tensor: | |
"""Generate response using CSM-1B model""" | |
generator = app.state.generator | |
# Validate model availability | |
if generator is None: | |
raise RuntimeError("TTS model not loaded") | |
# Setup context segments | |
segments = [] | |
for ctx in context: | |
if 'speaker' not in ctx or 'text' not in ctx or 'audio' not in ctx: | |
continue | |
# Decode base64 audio | |
audio_data = base64.b64decode(ctx['audio']) | |
# Convert to tensor | |
audio_tensor = convert_audio_data(audio_data) | |
# Create segment | |
segments.append({ | |
"speaker": ctx['speaker'], | |
"text": ctx['text'], | |
"audio": audio_tensor | |
}) | |
# Format text for better voice consistency | |
from app.prompt_engineering import format_text_for_voice | |
# Determine voice name from speaker_id | |
voice_names = ["alloy", "echo", "fable", "onyx", "nova", "shimmer"] | |
voice_name = voice_names[speaker_id] if 0 <= speaker_id < len(voice_names) else "alloy" | |
formatted_text = format_text_for_voice(text, voice_name) | |
# Generate audio with context | |
audio = generator.generate( | |
text=formatted_text, | |
speaker=speaker_id, | |
context=segments, | |
max_audio_length_ms=10000, # 10 seconds max for low latency | |
temperature=0.65, # Lower temperature for more stable output | |
topk=40, | |
) | |
# Process audio for better quality | |
from app.voice_enhancement import process_generated_audio | |
processed_audio = process_generated_audio( | |
audio, | |
voice_name, | |
generator.sample_rate, | |
text | |
) | |
return processed_audio | |
def is_silence(audio_data: bytes, threshold=SILENCE_THRESHOLD) -> bool: | |
"""Check if audio is silence""" | |
with io.BytesIO(audio_data) as buf: | |
try: | |
audio = AudioSegment.from_file(buf) | |
# Get RMS (root mean square) amplitude | |
rms = audio.rms | |
return rms < threshold | |
except: | |
# If can't process, assume not silent | |
return False | |
async def websocket_conversation(websocket: WebSocket, client_id: str): | |
"""WebSocket endpoint for real-time audio conversation""" | |
await manager.connect(websocket, client_id) | |
# Get access to app state through the websocket | |
app = websocket.app | |
# Validate model availability | |
if not hasattr(app.state, "generator") or app.state.generator is None: | |
await manager.send_message(client_id, MessageType.ERROR, | |
{"message": "TTS model not available"}) | |
manager.disconnect(client_id) | |
return | |
# Initialize audio buffer and state | |
audio_buffer = io.BytesIO() | |
is_speaking = False | |
silence_start = None | |
try: | |
# Tell client we're ready | |
await manager.send_message(client_id, MessageType.STATUS, | |
{"status": "ready", "message": "Connection established"}) | |
# Process messages | |
async for message in websocket.iter_json(): | |
message_type = message.get("type") | |
if message_type == "audio_chunk": | |
# Get audio data | |
audio_data = base64.b64decode(message["data"]) | |
# Check if silence or speech | |
current_is_silence = is_silence(audio_data) | |
# Handle silence detection for end of speech | |
if current_is_silence: | |
if not silence_start: | |
silence_start = time.time() | |
elif time.time() - silence_start > MIN_SILENCE_DURATION and is_speaking: | |
# End of speech detected | |
is_speaking = False | |
# Get audio from buffer | |
audio_buffer.seek(0) | |
full_audio = audio_buffer.read() | |
# Reset buffer | |
audio_buffer = io.BytesIO() | |
# Process the complete audio asynchronously | |
asyncio.create_task(process_complete_audio( | |
app, client_id, full_audio | |
)) | |
# Notify client of end of speech | |
await manager.send_message(client_id, MessageType.STOP_SPEAKING, {}) | |
else: | |
# Reset silence detection on new speech | |
silence_start = None | |
# Start of speech if not already speaking | |
if not is_speaking: | |
is_speaking = True | |
await manager.send_message(client_id, MessageType.START_SPEAKING, {}) | |
# Add chunk to buffer if speaking | |
if is_speaking: | |
audio_buffer.write(audio_data) | |
elif message_type == "end_audio": | |
# Explicit end of audio from client | |
if audio_buffer.tell() > 0: | |
# Get audio from buffer | |
audio_buffer.seek(0) | |
full_audio = audio_buffer.read() | |
# Reset buffer | |
audio_buffer = io.BytesIO() | |
is_speaking = False | |
# Process the complete audio asynchronously | |
asyncio.create_task(process_complete_audio( | |
app, client_id, full_audio | |
)) | |
elif message_type == "set_voice": | |
# Set the voice for the response | |
voice = message.get("voice", "alloy") | |
# Map voice string to speaker ID | |
voice_to_speaker = {"alloy": 0, "echo": 1, "fable": 2, "onyx": 3, "nova": 4, "shimmer": 5} | |
speaker_id = voice_to_speaker.get(voice, 0) | |
# Store in client state | |
manager.set_voice_preference(client_id, speaker_id) | |
# Send confirmation to client | |
await manager.send_message(client_id, MessageType.STATUS, | |
{"status": "voice_set", "voice": voice, "speaker_id": speaker_id}) | |
elif message_type == "clear_context": | |
# Clear the conversation context | |
if client_id in manager.conversation_contexts: | |
manager.conversation_contexts[client_id] = [] | |
await manager.send_message(client_id, MessageType.STATUS, | |
{"status": "context_cleared"}) | |
except WebSocketDisconnect: | |
logger.info(f"Client {client_id} disconnected") | |
except Exception as e: | |
logger.error(f"Error in websocket conversation: {e}", exc_info=True) | |
try: | |
await manager.send_message(client_id, MessageType.ERROR, | |
{"message": str(e)}) | |
except: | |
pass | |
finally: | |
manager.disconnect(client_id) | |
async def process_complete_audio(app, client_id: str, audio_data: bytes): | |
"""Process complete audio chunk from WebSocket""" | |
try: | |
# Transcribe audio | |
transcription = await transcribe_audio(audio_data) | |
# Get the text | |
text = transcription.get("text", "").strip() | |
# Send transcription to client | |
await manager.send_message(client_id, MessageType.TRANSCRIPT, | |
{"text": text, "segments": transcription.get("segments", [])}) | |
# Skip if empty text | |
if not text: | |
return | |
# Add user message to context (user is always speaker 0) | |
manager.add_to_context(client_id, 0, text, audio_data) | |
# Get current context | |
context = manager.get_context(client_id) | |
# Generate response | |
voice_id = manager.get_voice_preference(client_id) | |
response_audio = await generate_response(app, text, voice_id, context) | |
# Convert to bytes | |
response_bytes = convert_tensor_to_wav_bytes(response_audio) | |
response_base64 = base64.b64encode(response_bytes).decode('utf-8') | |
# Send response to client | |
await manager.send_message(client_id, MessageType.RESPONSE, { | |
"audio": response_base64, | |
"speaker_id": voice_id | |
}) | |
# Add assistant response to context | |
manager.add_to_context(client_id, voice_id, text, response_audio) | |
except Exception as e: | |
logger.error(f"Error processing audio: {e}", exc_info=True) | |
await manager.send_message(client_id, MessageType.ERROR, | |
{"message": f"Error processing audio: {str(e)}"}) |